To process the huge mass of images generated by camera traps installed around the mountains, CREA Mont-Blanc developed a hybrid system using the expertise of researchers, machine-learning, and citizen science. Currently in the experimentation and developmental phase, it has already allowed us to obtain initial results. Lire l’article en français ici !

Acquiring large amounts of data is great, but being able to process and analyze them is even better! And that’s the big challenge posed by the use of camera traps to study alpine fauna. To quantify this challenge: the 36 devices which CREA Mont-Blanc installed on the Orchamp plots take 150,000 images per year, which represent to date more than 400,000 photos that must be stored and then most importantly deciphered, classified and archived.
Detecting and identifying animals
Processing the data specifically means detecting the presence or absence of an animal in each photo and then identifying the species to which it belongs. We use various methods to accomplish this goal. The first consists of manual observation by our researchers of all of the images; however at a rate of 2,000 images per hour by an expert eye, this solution takes a considerable amount of time, and is difficult to fit into the schedule dictated by the human and financial resources available at CREA Mont-Blanc.
For this reason, we are turning to an automated method of image analysis using machine-learning, developed by our partner Devoteam Revolve. With this solution, the computer itself identifies the animals present in the photos. In order to achieve this, it must learn to interpret the images and classify them, which requires a very large number of photos. “Obviously, a computer does not know what a chamois is. We give it an initial reference dataset which contains relevant information. The larger and more varied this dataset, the better the computer learns, that’s how machine learning works” explains Anne Delestrade, director of CREA Mont-Blanc.
This processing method, although still in its testing phase, has already produced encouraging results. For example, the simple task of identifying the cases where there is nothing to see makes it possible to immediately eliminate two thirds of the images where the camera was triggered by something other than the presence of an animal (snow, wind moving a branch…).
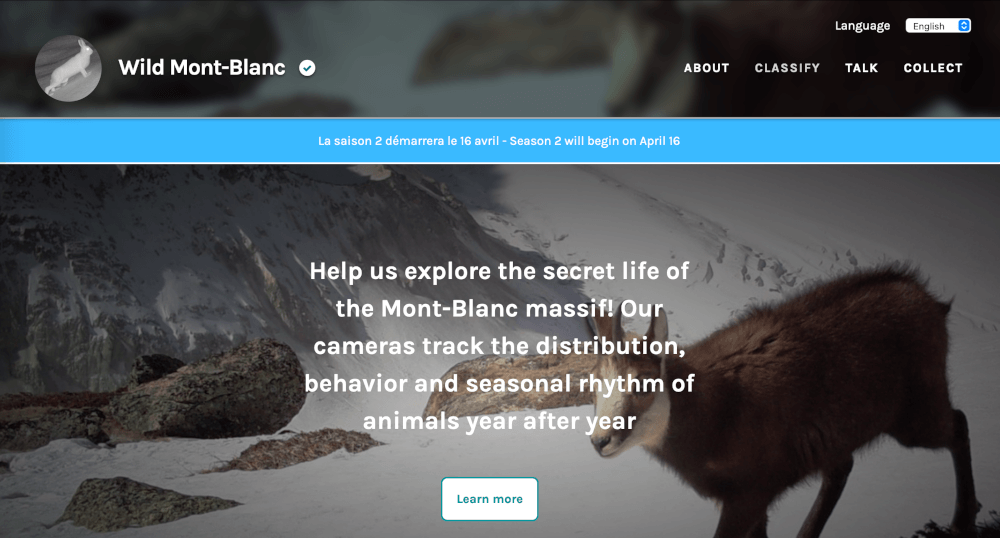
The value of reaching out to the public
Since last year, the Wild Mont-Blanc platform allows all those who wish to participate in the processing of images online to do so from their own computers: 5,500 volunteers performed more than a million classifications in 2020, and a new “season” will launch in April. This participatory method, which corresponds well with the citizen science hands-on approach of CREA Mont-Blanc, encourages education and awareness of scientific research to a wide audience.
Above all, this method contributes to the refinement of the machine-learning models. The participants can improve the accuracy of the identification in certain photos which the computer will not have clearly identified, or not identified well. They can go even further than the machine and describe the behavior of the animals: “It will be difficult for the computer to discern whether an animal is eating, moving or resting. This information is of interest to us so that we know whether the animals are only passing through certain environments or if they are feeding there,” continues Anne. Like machine-learning, public participation must be evaluated in order to analyze any errors and determine the optimal number of participants for obtaining somewhat accurate data. The current phase consists of refining the model to find a system which mixes these different methods.
Initial results of the analysis
Once the data have been processed, they still need to be analyzed. This stage requires a huge amount of statistical work on the raw data in order to obtain a relative abundance index, indicative of the presence of different species at each site. This index takes into account detection rates, which are themselves impacted by different observation biases linked to the behavior of animals and to variations in the environment. This work is in progress within the framework of the study on large herbivores.
The initial results give indications of how different species visit the observed environments according to the seasons and their daily activity. The roe deer is, for example, usually identified in the forest, the red deer mostly in the forest and in the heaths during the spring and summer, and the chamois is present everywhere. This confirms that there are overlaps between the species, which is one of the questions that we are investigating as part of the study. Now, we have to interpret the data more specifically, in particular by cross-referencing them with information on vegetation (such as a greenness index) and snow cover, and to see how animal species adapt to changes in their environment over time.
Read the article introducing our observations with camera traps: “How Camera Traps Significantly Increase our Means of Observation”
Read the article about Season 1 of Wild Mont-Blanc
Visit the Wild Mont-Blanc platform and participate in Season 2 in April 2021.
Translated from French into English by Anna Saviano and Warren Galloway.
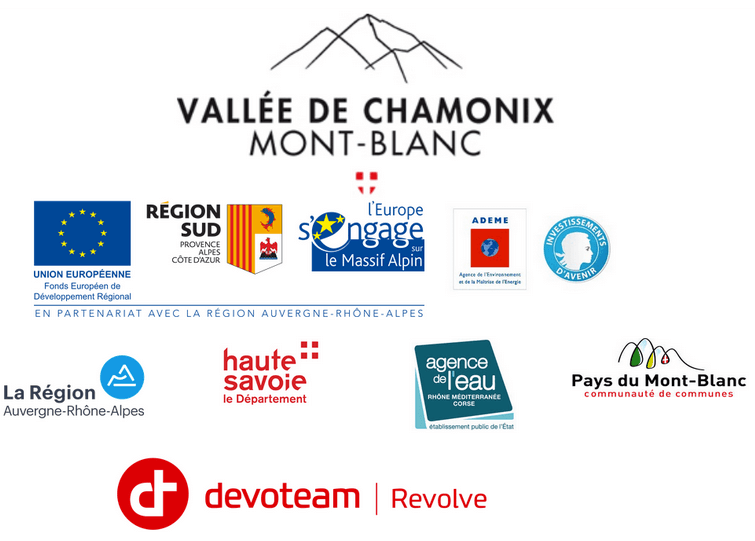
Leave a Reply